@inproceedings{zulqarnain2025, author = {Zulqarnain, Ammar and Buckelew, Jacob and Talusan, Jose Paolo and Mukhopadhyay, Ayan and Dubey, Abhishek}, booktitle = {2025 IEEE International Conference on Smart Computing (SMARTCOMP)}, title = {TRACE: Traffic Response Anomaly Capture Engine for Localization of Traffic Incidents}, year = {2025}, month = jun, contribution = {lead} }
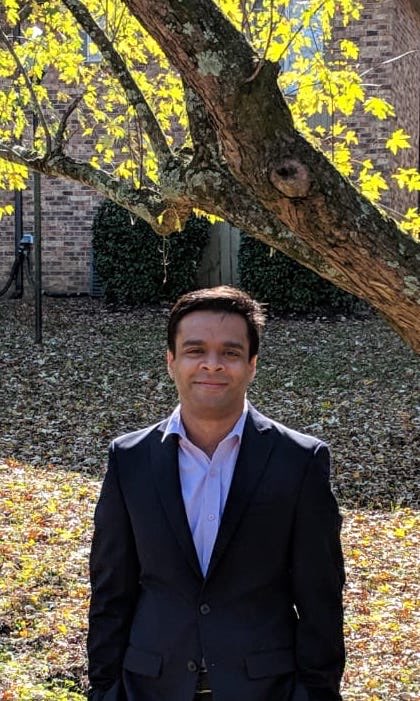
Dr. Ayan Mukhopadhyay is a Senior Research Scientist in the Department of Electrical Engineering and Computer Science at Vanderbilt University, USA. Prior to this, he was a Post-Doctoral Research Fellow at the Stanford Intelligent Systems Lab at Stanford University, USA. He was awarded the 2019 CARS post-doctoral fellowship by the Center of Automotive Research at Stanford (CARS). Before joining Stanford, he completed his Ph.D. at Vanderbilt University’s Computational Economics Research Lab, and his doctoral thesis was nominated for the Victor Lesser Distinguished Dissertation Award 2020. His research interests include multi-agent systems, robust machine learning, and decision-making under uncertainty applied to the intersection of CPS and smart cities. His work has been published in several top-tier AI and CPS conferences like AAMAS, UAI, and ICCPS. His work on creating proactive emergency response pipelines has been covered in the government technology magazine, won the best paper award at ICLR’s AI for Social Good Workshop, and covered in multiple smart city symposiums.
Dr. Ayan Mukhopadhyay Publications with ScopeLab
- A. Zulqarnain, J. Buckelew, J. P. Talusan, A. Mukhopadhyay, and A. Dubey, TRACE: Traffic Response Anomaly Capture Engine for Localization of Traffic Incidents, in 2025 IEEE International Conference on Smart Computing (SMARTCOMP), 2025.
Effective traffic incident management is critical for road safety and operational efficiency. Yet, many transportation agencies rely on reactionary methods, where incidents are reported by human agents and managed through rule- based frameworks like traditional Traffic Incident Management (TIM) systems. However, these are vulnerable to human error, oversight, and delays during high-stress conditions. Although recent initiatives incorporating real-time sensor data for cor- ridor monitoring and enhanced roadway information systems represent strides toward modernization, these systems often still require substantial human intervention. Recent advancements in graph-based deep learning models offer promising potential for addressing the limitations of traditional methods. While state- of-the-art models exist, the complexities of incident localization within dynamic and interconnected road networks, along with limited availability of high-quality labeled data and variability in real-time traffic measurements, are still open challenges. To address these, we propose the Traffic Response Anomaly Capture Engine (TRACE), a novel approach that combines graph neural networks, transformers, and probabilistic normalizing flows to accurately detect and localize traffic anomalies in real time. TRACE captures spatial-temporal dependencies, manages data uncertainty, and enhances automation, supporting more precise and timely incident localization. Our approach is validated on real-world traffic data and improved incident localization by 0.6 miles (17%) than SOTA methods while maintaining similar incident detection accuracy and mean detection delay.
- A. Dubey, M. Wilbur, A. Mukhopadhyay, and A. Laszka, Forecasting energy consumption in a mixed-vehicle fleet, US Patent App. 18/708,438. Jan-2025.
@misc{dubey2025forecasting, author = {Dubey, Abhishek and Wilbur, Michael and Mukhopadhyay, Ayan and Laszka, Aron}, month = jan, title = {Forecasting energy consumption in a mixed-vehicle fleet}, year = {2025}, journal = {US Patent App. 18/708,438}, url = {https://patents.google.com/patent/US20250030766A1/en} }
- Z. An, X. Wang, H. Baier, Z. Chen, A. Dubey, T. T. Johnson, J. Sprinkle, A. Mukhopadhyay, and M. Ma, LogiEx: Integrating Formal Logic and Large Language Model for Explainable Planning, in Proceedings of the 24th International Conference on Autonomous Agents and Multiagent Systems (AAMAS 2025), 2025.
@inproceedings{an2025logiex, author = {An, Ziyan and Wang, Xia and Baier, Hendrik and Chen, Zirong and Dubey, Abhishek and Johnson, Taylor T. and Sprinkle, Jonathan and Mukhopadhyay, Ayan and Ma, Meiyi}, booktitle = {Proceedings of the 24th International Conference on Autonomous Agents and Multiagent Systems (AAMAS 2025)}, title = {LogiEx: Integrating Formal Logic and Large Language Model for Explainable Planning}, year = {2025}, note = {Extended Abstract}, acceptance = {40}, contribution = {colab} }
- N. S. Keplinger, B. Luo, I. Bektas, Y. Zhang, K. H. Wray, A. Laszka, A. Dubey, and A. Mukhopadhyay, NS-Gym: Open-Source Simulation Environments and Benchmarks for Non-Stationary Markov Decision Processes. 2025.
@misc{keplinger2025nsgymopensourcesimulationenvironments, author = {Keplinger, Nathaniel S. and Luo, Baiting and Bektas, Iliyas and Zhang, Yunuo and Wray, Kyle Hollins and Laszka, Aron and Dubey, Abhishek and Mukhopadhyay, Ayan}, title = {NS-Gym: Open-Source Simulation Environments and Benchmarks for Non-Stationary Markov Decision Processes}, year = {2025}, archiveprefix = {arXiv}, contribution = {colab}, eprint = {2501.09646}, primaryclass = {cs.AI}, url = {https://arxiv.org/abs/2501.09646} }
In many real-world applications, agents must make sequential decisions in environments where conditions are subject to change due to various exogenous factors. These non-stationary environments pose significant challenges to traditional decision-making models, which typically assume stationary dynamics. Non-stationary Markov decision processes (NS-MDPs) offer a framework to model and solve decision problems under such changing conditions. However, the lack of standardized benchmarks and simulation tools has hindered systematic evaluation and advance in this field. We present NS-Gym, the first simulation toolkit designed explicitly for NS-MDPs, integrated within the popular Gymnasium framework. In NS-Gym, we segregate the evolution of the environmental parameters that characterize non-stationarity from the agent’s decision-making module, allowing for modular and flexible adaptations to dynamic environments. We review prior work in this domain and present a toolkit encapsulating key problem characteristics and types in NS-MDPs. This toolkit is the first effort to develop a set of standardized interfaces and benchmark problems to enable consistent and reproducible evaluation of algorithms under non-stationary conditions. We also benchmark six algorithmic approaches from prior work on NS-MDPs using NS-Gym. Our vision is that NS-Gym will enable researchers to assess the adaptability and robustness of their decision-making algorithms to non-stationary conditions.
- A. Khanna, F. Liu, S. Gupta, S. Pavia, A. Mukhopadhyay, and A. Dubey, PDPTW-DB: MILP-Based Offline Route Planning for PDPTW with Driver Breaks, in Proceedings of the 26th International Conference on Distributed Computing and Networking, New York, NY, USA, 2025, pp. 73–83.
@inproceedings{khanna2025driverbreaks, author = {Khanna, Agrima and Liu, Fangqi and Gupta, Samir and Pavia, Sophie and Mukhopadhyay, Ayan and Dubey, Abhishek}, booktitle = {Proceedings of the 26th International Conference on Distributed Computing and Networking}, title = {PDPTW-DB: MILP-Based Offline Route Planning for PDPTW with Driver Breaks}, year = {2025}, address = {New York, NY, USA}, pages = {73--83}, acceptance = {32}, publisher = {Association for Computing Machinery}, series = {ICDCN '25}, category = {other}, contribution = {lead}, doi = {10.1145/3700838.3700854}, isbn = {9798400710629}, keywords = {Microtransit, Vehicular Networks, Pickup and Delivery Problem with Time Windows, Driver Breaks, MILP, Optimization, Microtransit, Vehicular Networks, Pickup and Delivery Problem with Time Windows, Driver Breaks, MILP, Optimization}, numpages = {11}, url = {https://doi.org/10.1145/3700838.3700854} }
The Pickup and Delivery Problem with Time Windows (PDPTW) involves optimizing routes for vehicles to meet pickup and delivery requests within specific time constraints, a challenge commonly faced in logistics and transportation. Microtransit, a flexible and demand-responsive service using smaller vehicles within defined zones, can be effectively modeled as a PDPTW. Yet, the need for driver breaks—a key human constraint—is frequently overlooked in PDPTW solutions, despite being necessary for regulatory compliance. This study presents a novel mixed-integer linear programming formulation for the Pickup and Delivery Problem with Time Windows and Driver Breaks (PDPTW-DB). To the best of our knowledge this formulation is the first to consider mandatory periodic driver breaks within optimized Microtransit routes. The proposed model incorporates regulatory compliant break scheduling directly within the vehicle routing optimization framework. By considering driver break requirements as an integral component of the optimization process, rather than as a post-processing step, the model enables the generation of routes that respect hours of service regulations while minimizing operational costs. This integrated approach facilitates the generation of schedules that are operationally efficient and prioritize driver welfare through driver breaks. We work with a public transit agency from the southern USA, and highlight the specific nuances of driver break optimization, and present a Pickup and Delivery Problem with Time Windows formulation for optimizing Microtransit operations and scheduling driver breaks. We validate our approach using real-world data from the transit agency. Our results validate our formulation in producing cost-effective, and regulation-compliant solutions.
- F. Liu, R. Sen, J. Talusan, A. Pettet, A. Kandel, Y. Suzue, A. Mukhopadhyay, and A. Dubey, Reinforcement Learning-based Approach for Vehicle-to-Building Charging with Heterogeneous Agents and Long Term Rewards, in Proceedings of the 23rd Conference on Autonomous Agents and MultiAgent Systems, AAMAS 2025, Detroit, Michigan, Richland, SC, 2025.
@inproceedings{liu2024reinforcement, author = {Liu, Fangqi and Sen, Rishav and Talusan, Jose and Pettet, Ava and Kandel, Aaron and Suzue, Yoshinori and Mukhopadhyay, Ayan and Dubey, Abhishek}, booktitle = {Proceedings of the 23rd Conference on Autonomous Agents and MultiAgent Systems, {AAMAS} 2025, Detroit, Michigan}, title = {Reinforcement Learning-based Approach for Vehicle-to-Building Charging with Heterogeneous Agents and Long Term Rewards}, year = {2025}, address = {Richland, SC}, note = {nominated for best paper}, organization = {International Conference on Autonomous Agents and Multi-Agent Systems}, publisher = {International Foundation for Autonomous Agents and Multiagent Systems}, series = {AAMAS '25}, acceptance = {24.5}, category = {selective}, contribution = {lead}, location = {Detroit, Michigan} }
Strategic aggregation of electric vehicle batteries as energy reservoirs can optimize power grid demand, benefiting smart and connected communities, especially large office buildings that offer workplace charging. This involves optimizing charging and discharging to reduce peak energy costs and net peak demand, monitored over extended periods (e.g., a month), which involves making sequential decisions under uncertainty and delayed and sparse rewards, a continuous action space, and the complexity of ensuring generalization across diverse conditions. Existing algorithmic approaches, e.g., heuristic-based strategies, fall short in addressing real-time decision-making under dynamic conditions, and traditional reinforcement learning (RL) models struggle with large stateaction spaces, multi-agent settings, and the need for long-term reward optimization. To address these challenges, we introduce a novel RL framework that combines the Deep Deterministic Policy Gradient approach (DDPG) with action masking and efficient MILP-driven policy guidance. Our approach balances the exploration of continuous action spaces to meet user charging demands. Using real-world data from a major electric vehicle manufacturer, we show that our approach comprehensively outperforms many well-established baselines and several scalable heuristic approaches, achieving significant cost savings while meeting all charging requirements. Our results show that the proposed approach is one of the first scalable and general approaches to solving the V2B energy management challenge.
- B. Luo, A. Pettet, A. Laszka, A. Dubey, and A. Mukhopadhyay, Scalable Decision-Making In Stochastic Environments Through Learned Temporal Abstraction, in Proceedings of the 13th International Conference on Learning Representations, Singapore, 2025.
@inproceedings{luo2025scalable, author = {Luo, Baiting and Pettet, Ava and Laszka, Aron and Dubey, Abhishek and Mukhopadhyay, Ayan}, booktitle = {Proceedings of the 13th International Conference on Learning Representations, Singapore}, title = {Scalable Decision-Making In Stochastic Environments Through Learned Temporal Abstraction}, year = {2025}, organization = {International Conference on Learning Representations}, acceptance = {32.8}, category = {selective}, contribution = {colab} }
Sequential decision-making in high-dimensional continuous action spaces, particularly in stochastic environments, faces significant computational challenges. We explore this challenge in the traditional offline RL setting, where an agent must learn how to make decisions based on data collected through a stochastic behavior policy. We present Latent Macro Action Planner (L-MAP), which addresses this challenge by learning a set of temporally extended macro-actions through a state-conditional Vector Quantized Variational Autoencoder (VQ-VAE), effectively reducing action dimensionality. L-MAP employs a (separate) learned prior model that acts as a latent transition model and allows efficient sampling of plausible actions. During planning, our approach accounts for stochasticity in both the environment and the behavior policy by using Monte Carlo tree search (MCTS). In offline RL settings, including stochastic continuous control tasks, L-MAP efficiently searches over discrete latent actions to yield high expected returns. Empirical results demonstrate that L-MAP maintains low decision latency despite increased action dimensionality. Notably, across tasks ranging from continuous control with inherently stochastic dynamics to high-dimensional robotic hand manipulation, L-MAP significantly outperforms existing model-based methods and performs on par with strong model-free actor-critic baselines, highlighting the effectiveness of the proposed approach in planning in complex and stochastic environments with high-dimensional action spaces.
- R. Sen, Y. Zhang, F. Liu, J. P. Talusan, A. Pettet, Y. Suzue, A. Mukhopadhyay, and A. Dubey, Online Decision-Making Under Uncertainty for Vehicle-to-Building Systems, in Proceedings of the ACM/IEEE 16th International Conference on Cyber-Physical Systems (ICCPS), New York, NY, USA, 2025.
@inproceedings{sen2025iccps, author = {Sen, Rishav and Zhang, Yunuo and Liu, Fangqi and Talusan, Jose Paolo and Pettet, Ava and Suzue, Yoshinori and Mukhopadhyay, Ayan and Dubey, Abhishek}, booktitle = {Proceedings of the ACM/IEEE 16th International Conference on Cyber-Physical Systems (ICCPS)}, title = {Online Decision-Making Under Uncertainty for Vehicle-to-Building Systems}, year = {2025}, address = {New York, NY, USA}, publisher = {Association for Computing Machinery}, series = {ICCPS '25}, acceptance = {28.4}, category = {selective}, contribution = {lead}, location = {California, USA}, numpages = {10}, ranking = {rank1} }
Vehicle-to-building (V2B) systems combine physical infrastructure such as smart buildings and electric vehicles (EVs) connected to chargers at the building, with digital control mechanisms to manage energy use. By utilizing EVs as flexible energy reservoirs, buildings can dynamically charge and discharge EVs to effectively manage energy usage, and reduce costs under time-variable pricing and demand charge policies. This setup leads to the V2B optimization problem, where buildings coordinate EV charging and discharging to minimize total electricity costs while meeting users’ charging requirements. However, the V2B optimization problem is difficult due to: 1) fluctuating electricity pricing, which includes both energy charges (/kWh) and demand charges (/kW); 2) long planning horizons (usually over 30 days); 3) heterogeneous chargers with differing charging rates, controllability, and directionality (unidirectional or bidirectional); and 4) user-specific battery levels at departure to ensure user requirements are met. While existing approaches often model this setting as a single-shot combinatorial optimization problem, we highlight critical limitations in prior work and instead model the V2B optimization problem as a Markov decision process, i.e., a stochastic control process. Solving the resulting MDP is challenging due to the large state and action spaces. To address the challenges of the large state space, we leverage online search, and we counter the action space by using domain-specific heuristics to prune unpromising actions. We validate our approach in collaboration with an EV manufacturer and a smart building operator in California, United States, showing that the proposed framework significantly outperforms state-of-the-art methods.
- Y. Zhang, B. Luo, A. Mukhopadhyay, and A. Dubey, Observation Adaptation via Annealed Importance Resampling for POMDPs, in Proceedings of the 35th International Conference on Automated Planning and Scheduling (ICAPS), 2025.
@inproceedings{zhang2025observation, author = {Zhang, Yunuo and Luo, Baiting and Mukhopadhyay, Ayan and Dubey, Abhishek}, booktitle = {Proceedings of the 35th International Conference on Automated Planning and Scheduling ({ICAPS})}, title = {Observation Adaptation via Annealed Importance Resampling for {POMDPs}}, year = {2025}, note = {accepted as oral presentation}, publisher = {{AAAI} Press}, acceptance = {22.8}, category = {selective}, contribution = {lead}, url = {https://openreview.net/forum?id=KxxxyuOFg2&referrer=%5Bthe%20profile%20of%20Baiting%20Luo%5D(%2Fprofile%3Fid%3D~Baiting_Luo1)} }
Partially observable Markov decision processes (POMDPs) are a general mathematical model for sequential decision-making in stochastic environments under state uncertainty. POMDPs are often solved online, which enables the algorithm to adapt to new information in real time. Online solvers typically use bootstrap particle filters based on importance resampling for updating the belief distribution. Since directly sampling from the ideal state distribution given the latest observation and previous state is infeasible, particle filters approximate the posterior belief distribution by propagating states and adjusting weights through prediction and resampling steps. However, in practice, the importance resampling technique often leads to particle degeneracy and sample impoverishment when the state transition model poorly aligns with the posterior belief distribution, especially when the received observation is noisy. We propose an approach that constructs a sequence of bridge distributions between the state-transition and optimal distributions through iterative Monte Carlo steps, better accommodating noisy observations in online POMDP solvers. Our algorithm demonstrates significantly superior performance compared to state-of-the-art methods when evaluated across multiple challenging POMDP domains.
- N. M. Baig, L. Pedersen, X. Yang, A. Baranskaya, L. Atkins, K. Wray, A. Dubey, G. Pettet, A. Mukhopadhyay, J. P. Talusan, and others, Electric vehicle charging control device, US Patent App. 18/309,772. Oct-2024.
@misc{baig2024electric, author = {Baig, Najamuddin Mirza and Pedersen, Liam and Yang, Xin and Baranskaya, Anna and Atkins, Lance and Wray, Kyle and Dubey, Abhishek and Pettet, Geoffrey and Mukhopadhyay, Ayan and Talusan, Jose Paolo and others}, month = oct, title = {Electric vehicle charging control device}, year = {2024}, journal = {US Patent App. 18/309,772}, url = {https://patents.google.com/patent/US20240359585A1/en} }
- S. Gupta, A. Khanna, J. P. Talusan, A. Said, D. Freudberg, A. Mukhopadhyay, and A. Dubey, A Graph Neural Network Framework for Imbalanced Bus Ridership Forecasting, in 2024 IEEE International Conference on Smart Computing (SMARTCOMP), 2024.
@inproceedings{samir2024smartcomp, author = {Gupta, Samir and Khanna, Agrima and Talusan, Jose Paolo and Said, Anwar and Freudberg, Dan and Mukhopadhyay, Ayan and Dubey, Abhishek}, booktitle = {2024 IEEE International Conference on Smart Computing (SMARTCOMP)}, title = {A Graph Neural Network Framework for Imbalanced Bus Ridership Forecasting}, year = {2024}, acceptance = {32.9}, month = jun, contribution = {lead} }
Public transit systems are paramount in lowering carbon emissions and reducing urban congestion for environmental sustainability. However, overcrowding has adverse effects on the quality of service, passenger experience, and overall efficiency of public transit causing a decline in the usage of public transit systems. Therefore, it is crucial to identify and forecast potential windows of overcrowding to improve passenger experience and encourage higher ridership. Predicting ridership is a complex task, due to the inherent noise of collected data and the sparsity of overcrowding events. Existing studies in predicting public transit ridership consider only a static depiction of bus networks. We address these issues by first applying a data processing pipeline that cleans noisy data and engineers several features for training. Then, we address sparsity by converting the network to a dynamic graph and using a graph convolutional network, incorporating temporal, spatial, and auto-regressive features, to learn generalizable patterns for each route. Finally, since conventional loss functions like categorical cross-entropy have limitations in addressing class imbalance inherent in ridership data, our proposed approach uses focal loss to refine the prediction focus on less frequent yet task-critical overcrowding instances. Our experiments, using real-world data from our partner agency, show that the proposed approach outperforms existing state-of-the-art baselines in terms of accuracy and robustness.
- J. P. Talusan, R. Sen, A. K. Ava Pettet, Y. Suzue, L. Pedersen, A. Mukhopadhyay, and A. Dubey, OPTIMUS: Discrete Event Simulator for Vehicle-to-Building Charging Optimization, in 2024 IEEE International Conference on Smart Computing (SMARTCOMP), 2024.
@inproceedings{talusan2024smartcomp, author = {Talusan, Jose Paolo and Sen, Rishav and Ava Pettet, Aaron Kandel and Suzue, Yoshinori and Pedersen, Liam and Mukhopadhyay, Ayan and Dubey, Abhishek}, booktitle = {2024 IEEE International Conference on Smart Computing (SMARTCOMP)}, title = {OPTIMUS: Discrete Event Simulator for Vehicle-to-Building Charging Optimization}, year = {2024}, month = jun, acceptance = {32.9}, contribution = {lead} }
The increasing popularity of electronic vehicles has spurred a demand for EV charging infrastructure. In the United States alone, over 160,000 public and private charging ports have been installed. This has stoked fear of potential grid issues in the future. Meanwhile, companies, specifically building owners are also seeing the opportunity to leverage EV batteries as energy stores to serve as buffers against the electric grid. The main idea is to influence and control charging behavior to provide a certain level of energy resiliency and demand responsiveness to the building from grid events while ensuring that they meet the demands of EV users. However, managing and co-optimizing energy requirements of EVs and cost-saving measures of building owners is a difficult task. First, user behavior and grid uncertainty contribute greatly to the potential effectiveness of different policies. Second, different charger configurations can have drastically different effects on the cost. Therefore, we propose a complete end-to-end discrete event simulator for vehicle-to-building charging optimization. This software is aimed at building owners and EV manufacturers such as Nissan, looking to deploy their charging stations with state-of-the-art optimization algorithms. We provide a complete solution that allows the owners to train, evaluate, introduce uncertainty, and benchmark policies on their datasets. Lastly, we discuss the potential for extending our work with other vehicle-to-grid deployments.
- Y. Senarath, A. Mukhopadhyay, H. Purohit, and A. Dubey, Designing a Human-centered AI Tool for Proactive Incident Detection Using Crowdsourced Data Sources to Support Emergency Response, Digit. Gov.: Res. Pract., vol. 5, no. 1, Mar. 2024.
@article{10.1145/3633784, author = {Senarath, Yasas and Mukhopadhyay, Ayan and Purohit, Hemant and Dubey, Abhishek}, journal = {Digit. Gov.: Res. Pract.}, title = {Designing a Human-centered AI Tool for Proactive Incident Detection Using Crowdsourced Data Sources to Support Emergency Response}, year = {2024}, month = mar, number = {1}, volume = {5}, address = {New York, NY, USA}, articleno = {9}, contribution = {colab}, doi = {10.1145/3633784}, issue_date = {March 2024}, keywords = {Emergency response, incident detection, human-centered ai tool, crowdsourcing}, numpages = {19}, publisher = {Association for Computing Machinery}, url = {https://doi.org/10.1145/3633784} }
Time of incident reporting is a critical aspect of emergency response. However, the conventional approaches to receiving incident reports have time delays. Non-traditional sources such as crowdsourced data present an opportunity to detect incidents proactively. However, detecting incidents from such data streams is challenging due to inherent noise and data uncertainty. Naively maximizing detection accuracy can compromise spatial-temporal localization of inferred incidents, hindering response efforts. This article presents a novel human-centered AI tool to address the above challenges. We demonstrate how crowdsourced data can aid incident detection while acknowledging associated challenges. We use an existing CROME framework to facilitate training and selection of best incident detection models, based on parameters suited for deployment. The human-centered AI tool provides a visual interface for exploring various measures to analyze the models for the practitioner’s needs, which could help the practitioners select the best model for their situation. Moreover, in this study, we illustrate the tool usage by comparing different models for incident detection. The experiments demonstrate that the CNN-based incident detection method can detect incidents significantly better than various alternative modeling approaches. In summary, this research demonstrates a promising application of human-centered AI tools for incident detection to support emergency response agencies.
- A. Sivagnanam, A. Pettet, H. Lee, A. Mukhopadhyay, A. Dubey, and A. Laszka, Multi-agent reinforcement learning with hierarchical coordination for emergency responder stationing, in Proceedings of the 41st International Conference on Machine Learning, 2024.
@inproceedings{10.5555/3692070.3693934, author = {Sivagnanam, Amutheezan and Pettet, Ava and Lee, Hunter and Mukhopadhyay, Ayan and Dubey, Abhishek and Laszka, Aron}, booktitle = {Proceedings of the 41st International Conference on Machine Learning}, title = {Multi-agent reinforcement learning with hierarchical coordination for emergency responder stationing}, year = {2024}, publisher = {JMLR.org}, series = {ICML'24}, articleno = {1864}, contribution = {colab}, acceptance = {27.5}, location = {Vienna, Austria}, numpages = {22}, url = {https://www.arxiv.org/pdf/2405.13205v1} }
An emergency responder management (ERM) system dispatches responders, such as ambulances, when it receives requests for medical aid. ERM systems can also proactively reposition responders between predesignated waiting locations to cover any gaps that arise due to the prior dispatch of responders or significant changes in the distribution of anticipated requests. Optimal repositioning is computationally challenging due to the exponential number of ways to allocate responders between locations and the uncertainty in future requests. The state-of-the-art approach in proactive repositioning is a hierarchical approach based on spatial decomposition and online Monte Carlo tree search, which may require minutes of computation for each decision in a domain where seconds can save lives. We address the issue of long decision times by introducing a novel reinforcement learning (RL) approach, based on the same hierarchical decomposition, but replacing online search with learning. To address the computational challenges posed by large, variable-dimensional, and discrete state and action spaces, we propose: (1) actor-critic based agents that incorporate transformers to handle variable-dimensional states and actions, (2) projections to fixed-dimensional observations to handle complex states, and (3) combinatorial techniques to map continuous actions to discrete allocations. We evaluate our approach using realworld data from two U.S. cities, Nashville, TN and Seattle, WA. Our experiments show that compared to the state of the art, our approach reduces computation time per decision by three orders of magnitude, while also slightly reducing average ambulance response time by 5 seconds.
- Z. An, H. Baier, A. Dubey, A. Mukhopadhyay, and M. Ma, Enabling MCTS Explainability for Sequential Planning Through Computation Tree Logic, in ECAI 2024 - 27th European Conference on Artificial Intelligence, 2024.
@inproceedings{an2024enablingmctsexplainabilitysequential, author = {An, Ziyan and Baier, Hendrik and Dubey, Abhishek and Mukhopadhyay, Ayan and Ma, Meiyi}, title = {Enabling MCTS Explainability for Sequential Planning Through Computation Tree Logic}, year = {2024}, archiveprefix = {arXiv}, booktitle = {{ECAI} 2024 - 27th European Conference on Artificial Intelligence}, contribution = {colab}, acceptance = {23}, eprint = {2407.10820}, location = {Santiago de Compostela, Spain}, primaryclass = {cs.AI}, url = {https://arxiv.org/abs/2407.10820} }
Monte Carlo tree search (MCTS) is one of the most capa- ble online search algorithms for sequential planning tasks, with sig- nificant applications in areas such as resource allocation and transit planning. Despite its strong performance in real-world deployment, the inherent complexity of MCTS makes it challenging to understand for users without technical background. This paper considers the use of MCTS in transportation routing services, where the algorithm is integrated to develop optimized route plans. These plans are required to meet a range of constraints and requirements simultaneously, fur- ther complicating the task of explaining the algorithm’s operation in real-world contexts. To address this critical research gap, we intro- duce a novel computation tree logic-based explainer for MCTS. Our framework begins by taking user-defined requirements and translat- ing them into rigorous logic specifications through the use of lan- guage templates. Then, our explainer incorporates a logic verifica- tion and quantitative evaluation module that validates the states and actions traversed by the MCTS algorithm. The outcomes of this anal- ysis are then rendered into human-readable descriptive text using a second set of language templates. The user satisfaction of our ap- proach was assessed through a survey with 82 participants. The re- sults indicated that our explanatory approach significantly outper- forms other baselines in user preference.
- B. Luo, Y. Zhang, A. Dubey, and A. Mukhopadhyay, Act as You Learn: Adaptive Decision-Making in Non-Stationary Markov Decision Processes, in Proceedings of the 23rd International Conference on Autonomous Agents and Multiagent Systems, Richland, SC, 2024, pp. 1301–1309.
@inproceedings{baiting2024AAMAS, author = {Luo, Baiting and Zhang, Yunuo and Dubey, Abhishek and Mukhopadhyay, Ayan}, booktitle = {Proceedings of the 23rd International Conference on Autonomous Agents and Multiagent Systems}, title = {Act as You Learn: Adaptive Decision-Making in Non-Stationary Markov Decision Processes}, year = {2024}, address = {Richland, SC}, pages = {1301–1309}, acceptance = {20}, publisher = {International Foundation for Autonomous Agents and Multiagent Systems}, series = {AAMAS '24}, contribution = {colab}, isbn = {9798400704864}, keywords = {monte carlo tree search, non-stationary environments, online planning, sequential decision-making}, location = {Auckland, New Zealand}, numpages = {9} }
A fundamental challenge in sequential decision-making is dealing with non-stationary environments, where exogenous environmental conditions change over time. Such problems are traditionally modeled as non-stationary Markov decision processes (NS-MDP). However, existing approaches for decision-making in NS-MDPs have two major shortcomings: first, they assume that the updated environmental dynamics at the current time are known (although future dynamics can change); and second, planning is largely pessimistic, i.e., the agent acts "safely” to account for the non-stationary evolution of the environment. We argue that both these assumptions are invalid in practice-updated environmental conditions are rarely known, and as the agent interacts with the environment, it can learn about the updated dynamics and avoid being pessimistic, at least in states whose dynamics it is confident about. We present a heuristic search algorithm called Adaptive Monte Carlo Tree Search (ADA-MCTS) that addresses these challenges. We show that the agent can learn the updated dynamics of the environment over time and then act as it learns, i.e., if the agent is in a region of the state space about which it has updated knowledge, it can avoid being pessimistic. To quantify "updated knowledge,” we disintegrate the aleatoric and epistemic uncertainty in the agent’s updated belief and show how the agent can use these estimates for decision-making. We compare the proposed approach with multiple state-of-the-art approaches in decision-making across multiple well-established open-source problems and empirically show that our approach is faster and more adaptive without sacrificing safety.
- A. Pani, H. Puppala, S. Jha, A. Gupta, A. Mukhopadhyay, and A. Dubey, Enhancing Urban Mobility with Aerial Ropeway Transit (ART): Future Accessibility Impacts of Multimodal Transit Expansion Scenarios, Transportation Research Record, vol. 0, no. 0, p. 03611981241270180, 2024.
@article{pani2024, author = {Pani, Agnivesh and Puppala, Harish and Jha, Shreepati and Gupta, Ankit and Mukhopadhyay, Ayan and Dubey, Abhishek}, journal = {Transportation Research Record}, title = {Enhancing Urban Mobility with Aerial Ropeway Transit (ART): Future Accessibility Impacts of Multimodal Transit Expansion Scenarios}, year = {2024}, number = {0}, pages = {03611981241270180}, volume = {0}, contribution = {colab}, doi = {10.1177/03611981241270180}, url = {https://doi.org/10.1177/03611981241270180} }
Aerial ropeway transit (ART) systems are emerging alternatives to augment existing transit systems in congested cities in the Global South, especially in urban areas with limited transit coverage because of road width constraints or topography. Integration of aerial cable car stations to an existing transit network can improve the overall accessibility of various population segments with significant positive benefits in relation to reducing transport-related social exclusion. This study evaluated the impact of introducing ART in the city of Varanasi (India) and assessed the spatial accessibility improvements to critical facility locations such as heritage sites, educational institutions, hospitals, and employment centers. Several multimodal transit expansion scenarios were considered in this study and the potential benefits of each case were quantified using the two-step floating catchment area (2SFCA) method. A multi-criteria decision-making (MCDM) approach was subsequently employed for identifying the optimal locations of ART stops. Microlevel analysis findings suggest that the mean accessibility values could increase up to 10.92% in the first phase of the ART implementation, which could subsequently increase to 24.7% and 49.8% for the subsequent transit expansion scenarios. The study also investigated the Varanasi ART DPR prepared by Varanasi Development Authority (VDA) and showed that a significant increase of 16% in accessibility levels could be achieved if optimal stop locations identified in this study were implemented. The proposed two-step (2SFCA+MCDM) method for identifying the optimal locations of ART stations in a multimodal transit network is expected to be an effective tool for transit system redesign using place-based accessibility measures.
- S. Pavia, D. Rogers, A. Sivagnanam, M. Wilbur, D. Edirimanna, Y. Kim, P. Pugliese, S. Samaranayake, A. Laszka, A. Mukhopadhyay, and A. Dubey, Deploying mobility-on-demand for all by optimizing paratransit services, in Proceedings of the Thirty-Third International Joint Conference on Artificial Intelligence, 2024.
@inproceedings{paviaIJCAI24AISG, author = {Pavia, Sophie and Rogers, David and Sivagnanam, Amutheezan and Wilbur, Michael and Edirimanna, Danushka and Kim, Youngseok and Pugliese, Philip and Samaranayake, Samitha and Laszka, Aron and Mukhopadhyay, Ayano and Dubey, Abhishek}, booktitle = {Proceedings of the Thirty-Third International Joint Conference on Artificial Intelligence}, title = {Deploying mobility-on-demand for all by optimizing paratransit services}, year = {2024}, series = {IJCAI '24}, articleno = {822}, contribution = {lead}, acceptance = {15}, doi = {10.24963/ijcai.2024/822}, isbn = {978-1-956792-04-1}, location = {Jeju, Korea}, numpages = {8}, url = {https://doi.org/10.24963/ijcai.2024/822} }
While on-demand ride-sharing services have become popular in recent years, traditional on-demand transit services cannot be used by everyone, e.g., people who use wheelchairs. Paratransit services, operated by public transit agencies, are a critical infrastructure that offers door-to-door transportation assistance for individuals who face challenges in using standard transit routes. However, with declining ridership and mounting financial pressure, public transit agencies in the USA struggle to operate existing services. We collaborate with a public transit agency from the southern USA, highlight the specific nuances of paratransit optimization, and present a vehicle routing problem formulation for optimizing paratransit. We validate our approach using real-world data from the transit agency, present results from an actual pilot deployment of the proposed approach in the city, and show how the proposed approach comprehensively outperforms existing approaches used by the transit agency. To the best of our knowledge, this work presents one of the first examples of using open-source algorithmic approaches for paratransit optimization.
- S. Pavia, D. Rogers, A. Sivagnanam, M. Wilbur, D. Edirimanna, Y. Kim, A. Mukhopadhyay, P. Pugliese, S. Samaranayake, A. Laszka, and A. Dubey, SmartTransit.AI: a dynamic paratransit and microtransit application, in Proceedings of the Thirty-Third International Joint Conference on Artificial Intelligence, 2024.
@inproceedings{paviaIJCAI24demo, author = {Pavia, Sophie and Rogers, David and Sivagnanam, Amutheezan and Wilbur, Michael and Edirimanna, Danushka and Kim, Youngseo and Mukhopadhyay, Ayan and Pugliese, Philip and Samaranayake, Samitha and Laszka, Aron and Dubey, Abhishek}, booktitle = {Proceedings of the Thirty-Third International Joint Conference on Artificial Intelligence}, title = {SmartTransit.AI: a dynamic paratransit and microtransit application}, year = {2024}, series = {IJCAI '24}, articleno = {1028}, contribution = {lead}, acceptance = {15}, doi = {10.24963/ijcai.2024/1028}, isbn = {978-1-956792-04-1}, location = {Jeju, Korea}, numpages = {4}, url = {https://doi.org/10.24963/ijcai.2024/1028} }
New rideshare and shared mobility services have transformed urban mobility in recent years. Such services have the potential to improve efficiency and reduce costs by allowing users to share rides in high-capacity vehicles and vans. Most transit agencies already operate various ridepooling services, including microtransit and paratransit. However, the objectives and constraints for implementing these services vary greatly between agencies and can be challenging. First, off-the-shelf ridepooling formulations must be adapted for real-world conditions and constraints. Second, the lack of modular and reusable software makes it hard to implement and evaluate new ridepooling algorithms and approaches in real-world settings. We demonstrate a modular on-demand public transportation scheduling software for microtransit and paratransit services. The software is aimed at transit agencies looking to incorporate state-of-the-art rideshare and ridepooling algorithms in their everyday operations. We provide management software for dispatchers and mobile applications for drivers and users and conclude with results from the demonstration in Chattanooga, TN.
- A. Pettet, Y. Zhang, B. Luo, K. Wray, H. Baier, A. Laszka, A. Dubey, and A. Mukhopadhyay, Decision Making in Non-Stationary Environments with Policy-Augmented Search, in Proceedings of the 23rd International Conference on Autonomous Agents and Multiagent Systems, Richland, SC, 2024, pp. 2417–2419.
@inproceedings{pettet2024decision, author = {Pettet, Ava and Zhang, Yunuo and Luo, Baiting and Wray, Kyle and Baier, Hendrik and Laszka, Aron and Dubey, Abhishek and Mukhopadhyay, Ayan}, booktitle = {Proceedings of the 23rd International Conference on Autonomous Agents and Multiagent Systems}, title = {Decision Making in Non-Stationary Environments with Policy-Augmented Search}, year = {2024}, address = {Richland, SC}, acceptance = {36}, pages = {2417–2419}, publisher = {International Foundation for Autonomous Agents and Multiagent Systems}, series = {AAMAS '24}, contribution = {lead}, isbn = {9798400704864}, note = {extended abstract}, keywords = {mcts, non-stationary environments, sequential decision-making}, location = {Auckland, New Zealand}, numpages = {3} }
Sequential decision-making is challenging in non-stationary environments, where the environment in which an agent operates can change over time. Policies learned before execution become stale when the environment changes, and relearning takes time and computational effort. Online search, on the other hand, can return sub-optimal actions when there are limitations on allowed runtime. In this paper, we introduce Policy-Augmented Monte Carlo tree search (PA-MCTS), which combines action-value estimates from an out-of-date policy with an online search using an up-to-date model of the environment. We prove several theoretical results about PA-MCTS. We also compare and contrast our approach with AlphaZero, another hybrid planning approach, and Deep Q Learning on several OpenAI Gym environments and show that PA-MCTS outperforms these baselines.
- R. Sen, A. Sivagnanam, A. Laszka, A. Mukhopadhyay, and A. Dubey, Grid-Aware Charging and Operational Optimization for Mixed-Fleet Public Transit, in 2024 IEEE 27th International Conference on Intelligent Transportation Systems (ITSC), 2024.
@inproceedings{rishavITSC2024, author = {Sen, Rishav and Sivagnanam, Amutheezan and Laszka, Aron and Mukhopadhyay, Ayan and Dubey, Abhishek}, booktitle = {2024 IEEE 27th International Conference on Intelligent Transportation Systems (ITSC)}, title = {Grid-Aware Charging and Operational Optimization for Mixed-Fleet Public Transit}, year = {2024}, contribution = {lead}, keywords = {Mixed transit fleet, electrification, dynamic pricing, hierarchical MILP} }
The rapid growth of urban populations and the increasing need for sustainable transportation solutions have prompted a shift towards electric buses in public transit systems. However, the effective management of mixed fleets consisting of both electric and diesel buses poses significant operational chal- lenges. One major challenge is coping with dynamic electricity pricing, where charging costs vary throughout the day. Transit agencies must optimize charging assignments in response to such dynamism while accounting for secondary considerations such as seating constraints. This paper presents a comprehensive mixed-integer linear programming (MILP) model to address these challenges by jointly optimizing charging schedules and trip assignments for mixed (electric and diesel bus) fleets while considering factors such as dynamic electricity pricing, vehicle capacity, and route constraints. We address the potential computational intractability of the MILP formulation, which can arise even with relatively small fleets, by employing a hierarchical approach tailored to the fleet composition. By using real-world data from the city of Chattanooga, Tennessee, USA, we show that our approach can result in significant savings in the operating costs of the mixed transit fleets.
- C. Han, J. P. Talusan, D. Freudberg, A. Mukhopadhyay, A. Dubey, and A. Laszka, Forecasting and Mitigating Disruptions in Public Bus Transit Services, in Proceedings of the 23rd Conference on Autonomous Agents and MultiAgent Systems, AAMAS 2024, Auckland, New Zealand, Richland, SC, 2024.
@inproceedings{talusan2024AAMAS, author = {Han, Chaeeun and Talusan, Jose Paolo and Freudberg, Dan and Mukhopadhyay, Ayan and Dubey, Abhishek and Laszka, Aron}, booktitle = {Proceedings of the 23rd Conference on Autonomous Agents and MultiAgent Systems, {AAMAS} 2024, Auckland, New Zealand}, title = {Forecasting and Mitigating Disruptions in Public Bus Transit Services}, year = {2024}, address = {Richland, SC}, publisher = {International Foundation for Autonomous Agents and Multiagent Systems}, series = {AAMAS '24}, acceptance = {20}, contribution = {colab}, keywords = {Public transportation, Data-driven optimization, Disruption forecasting, Simulation, Metaheuristic optimization}, location = {Auckland, New Zealand}, numpages = {9} }
Public transportation systems often suffer from unexpected fluctuations in demand and disruptions, such as mechanical failures and medical emergencies. These fluctuations and disruptions lead to delays and overcrowding, which are detrimental to the passengers’ experience and to the overall performance of the transit service. To proactively mitigate such events, many transit agencies station substitute (reserve) vehicles throughout their service areas, which they can dispatch to augment or replace vehicles on routes that suffer overcrowding or disruption. However, determining the optimal locations where substitute vehicles should be stationed is a challenging problem due to the inherent randomness of disruptions and due to the combinatorial nature of selecting locations across a city. In collaboration with the transit agency of a mid-size U.S. city, we address this problem by introducing data-driven statistical and machine-learning models for forecasting disruptions and an effective randomized local-search algorithm for selecting locations where substitute vehicles are to be stationed. Our research demonstrates promising results in proactive disruption management, offering a practical and easily implementable solution for transit agencies to enhance the reliability of their services. Our results resonate beyond mere operational efficiency—by advancing proactive strategies, our approach fosters more resilient and accessible public transportation, contributing to equitable urban mobility and ultimately benefiting the communities that rely on public transportation the most.
- J. P. Talusan, C. Han, A. Mukhopadhyay, A. Laszka, D. Freudberg, and A. Dubey, An Online Approach to Solving Public Transit Stationing and Dispatch Problem, in Proceedings of the ACM/IEEE 15th International Conference on Cyber-Physical Systems (ICCPS), New York, NY, USA, 2024.
@inproceedings{talusan2024ICCPS, author = {Talusan, Jose Paolo and Han, Chaeeun and Mukhopadhyay, Ayan and Laszka, Aron and Freudberg, Dan and Dubey, Abhishek}, booktitle = {Proceedings of the ACM/IEEE 15th International Conference on Cyber-Physical Systems (ICCPS)}, title = {An Online Approach to Solving Public Transit Stationing and Dispatch Problem}, year = {2024}, address = {New York, NY, USA}, publisher = {Association for Computing Machinery}, series = {ICCPS '24}, contribution = {lead}, note = {Best paper award}, acceptance = {28.2}, location = {Hong Kong, China}, numpages = {10} }
Public bus transit systems provide critical transportation services for large sections of modern communities. On-time performance and maintaining the reliable quality of service is therefore very important. Unfortunately, disruptions caused by overcrowding, vehicular failures, and road accidents often lead to service performance degradation. Though transit agencies keep a limited number of vehicles in reserve and dispatch them to relieve the affected routes during disruptions, the procedure is often ad-hoc and has to rely on human experience and intuition to allocate resources (vehicles) to affected trips under uncertainty. In this paper, we describe a principled approach using non-myopic sequential decision procedures to solve the problem and decide (a) if it is advantageous to anticipate problems and proactively station transit buses near areas with high-likelihood of disruptions and (b) decide if and which vehicle to dispatch to a particular problem. Our approach was developed in partnership with the Metropolitan Transportation Authority for a mid-sized city in the USA and models the system as a semi-Markov decision problem (solved as a Monte-Carlo tree search procedure) and shows that it is possible to obtain an answer to these two coupled decision problems in a way that maximizes the overall reward (number of people served). We sample many possible futures from generative models, each is assigned to a tree and processed using root parallelization. We validate our approach using 3 years of data from our partner agency. Our experiments show that the proposed framework serves 2% more passengers while reducing deadhead miles by 40%.
- Y. Zhang, B. Luo, A. Mukhopadhyay, D. Stojcsics, D. Elenius, A. Roy, S. Jha, M. Maroti, X. Koutsoukos, G. Karsai, and A. Dubey, Shrinking POMCP: A Framework for Real-Time UAV Search and Rescue, in 2024 International Conference on Assured Autonomy (ICAA), 2024, pp. 48–57.
@inproceedings{zhang2024, author = {Zhang, Yunuo and Luo, Baiting and Mukhopadhyay, Ayan and Stojcsics, Daniel and Elenius, Daniel and Roy, Anirban and Jha, Susmit and Maroti, Miklos and Koutsoukos, Xenofon and Karsai, Gabor and Dubey, Abhishek}, booktitle = {2024 International Conference on Assured Autonomy (ICAA)}, title = {Shrinking POMCP: A Framework for Real-Time UAV Search and Rescue}, year = {2024}, pages = {48--57}, contribution = {lead}, doi = {10.1109/ICAA64256.2024.00016}, keywords = {Three-dimensional displays;Navigation;Markov decision processes;Urban areas;Probabilistic logic;Real-time systems;Trajectory;Maintenance;Time factors;Optimization;Search and Rescue;POMDP;MCTS} }
Efficient path optimization for drones in search and rescue operations faces challenges, including limited visibility, time constraints, and complex information gathering in urban environments. We present a comprehensive approach to optimize UAV-based search and rescue operations in neighborhood areas, utilizing both a 3D AirSim-ROS2 simulator and a 2D simulator. The path planning problem is formulated as a partially observable Markov decision process (POMDP), and we propose a novel "Shrinking POMCP" approach to address time constraints. In the AirSim environment, we integrate our approach with a probabilistic world model for belief maintenance and a neurosymbolic navigator for obstacle avoidance. The 2D simulator employs surrogate ROS2 nodes with equivalent functionality. We compare trajectories generated by different approaches in the 2D simulator and evaluate performance across various belief types in the 3D AirSim-ROS simulator. Experimental results from both simulators demonstrate that our proposed shrinking POMCP solution achieves significant improvements in search times compared to alternative methods, showcasing its potential for enhancing the efficiency of UAV-assisted search and rescue operations.
- B. Luo, S. Ramakrishna, A. Pettet, C. Kuhn, abhishek dubey, G. Karsai, and A. Mukhopadhyay, Dynamic Simplex: Balancing Safety and Performance in Autonomous Cyber Physical Systems, in Proceedings of the ACM/IEEE 14th International Conference on Cyber-Physical Systems (with CPS-IoT Week 2023), New York, NY, USA, 2023, pp. 177–186.
@inproceedings{baiting2023iccps, author = {Luo, Baiting and Ramakrishna, Shreyas and Pettet, Ava and Kuhn, Christopher and dubey, abhishek and Karsai, Gabor and Mukhopadhyay, Ayan}, booktitle = {Proceedings of the ACM/IEEE 14th International Conference on Cyber-Physical Systems (with CPS-IoT Week 2023)}, title = {Dynamic Simplex: Balancing Safety and Performance in Autonomous Cyber Physical Systems}, year = {2023}, address = {New York, NY, USA}, pages = {177--186}, publisher = {Association for Computing Machinery}, series = {ICCPS '23}, acceptance = {25.6}, contribution = {colab}, doi = {10.1145/3576841.3585934}, isbn = {9798400700361}, location = {San Antonio, TX, USA}, numpages = {10}, url = {https://doi.org/10.1145/3576841.3585934} }
Learning Enabled Components (LEC) have greatly assisted cyber-physical systems in achieving higher levels of autonomy. However, LEC’s susceptibility to dynamic and uncertain operating conditions is a critical challenge for the safety of these systems. Redundant controller architectures have been widely adopted for safety assurance in such contexts. These architectures augment LEC "performant" controllers that are difficult to verify with "safety" controllers and the decision logic to switch between them. While these architectures ensure safety, we point out two limitations. First, they are trained offline to learn a conservative policy of always selecting a controller that maintains the system’s safety, which limits the system’s adaptability to dynamic and non-stationary environments. Second, they do not support reverse switching from the safety controller to the performant controller, even when the threat to safety is no longer present. To address these limitations, we propose a dynamic simplex strategy with an online controller switching logic that allows two-way switching. We consider switching as a sequential decision-making problem and model it as a semi-Markov decision process. We leverage a combination of a myopic selector using surrogate models (for the forward switch) and a non-myopic planner (for the reverse switch) to balance safety and performance. We evaluate this approach using an autonomous vehicle case study in the CARLA simulator using different driving conditions, locations, and component failures. We show that the proposed approach results in fewer collisions and higher performance than state-of-the-art alternatives.
- J. Buckelew, S. Basumallik, V. Sivaramakrishnan, A. Mukhopadhyay, A. K. Srivastava, and A. Dubey, Synchrophasor Data Event Detection using Unsupervised Wavelet Convolutional Autoencoders, in 2023 IEEE International Conference on Smart Computing (SMARTCOMP), 2023, pp. 326–331.
@inproceedings{Buckelew2023, author = {Buckelew, Jacob and Basumallik, Sagnik and Sivaramakrishnan, Vasavi and Mukhopadhyay, Ayan and Srivastava, Anurag K. and Dubey, Abhishek}, booktitle = {2023 IEEE International Conference on Smart Computing (SMARTCOMP)}, title = {Synchrophasor Data Event Detection using Unsupervised Wavelet Convolutional Autoencoders}, year = {2023}, acceptance = {31}, pages = {326-331}, contribution = {lead}, doi = {10.1109/SMARTCOMP58114.2023.00080}, keywords = {Deep learning;Training;Event detection;Time series analysis;Power system stability;Feature extraction;Transformers;convolutional neural network;hardware-in-the-loop;unsupervised machine learning;phasor measurement units} }
Timely and accurate detection of events affecting the stability and reliability of power transmission systems is crucial for safe grid operation. This paper presents an efficient unsupervised machine-learning algorithm for event detection using a combination of discrete wavelet transform (DWT) and convolutional autoencoders (CAE) with synchrophasor phasor measurements. These measurements are collected from a hardware-in-the-loop testbed setup equipped with a digital real-time simulator. Using DWT, the detail coefficients of measurements are obtained. Next, the decomposed data is then fed into the CAE that captures the underlying structure of the transformed data. Anomalies are identified when significant errors are detected between input samples and their reconstructed outputs. We demonstrate our approach on the IEEE-14 bus system considering different events such as generator faults, line-to-line faults, line-to-ground faults, load shedding, and line outages simulated on a real-time digital simulator (RTDS). The proposed implementation achieves a classification accuracy of 97.7%, precision of 98.0%, recall of 99.5%, F1 Score of 98.7%, and proves to be efficient in both time and space requirements compared to baseline approaches.
- S. Pavia, J. C. M. Mori, A. Sharma, P. Pugliese, A. Dubey, S. Samaranayake, and A. Mukhopadhyay, Designing Equitable Transit Networks, ACM Conference on Equity and Access in Algorithms, Mechanisms, and Optimization (EAAMO). 2023.
@misc{pavia2023designing, author = {Pavia, Sophie and Mori, J. Carlos Martinez and Sharma, Aryaman and Pugliese, Philip and Dubey, Abhishek and Samaranayake, Samitha and Mukhopadhyay, Ayan}, title = {Designing Equitable Transit Networks}, year = {2023}, category = {poster}, acceptance = {16}, contribution = {lead}, journal = {ACM Conference on Equity and Access in Algorithms, Mechanisms, and Optimization (EAAMO)}, preprint = {https://arxiv.org/abs/2212.12007} }
public transit is an essential infrastructure enabling access to employment, healthcare, education, and recreational facilities. While accessibility to transit is important in general, some sections of the population depend critically on transit. However, existing public transit is often not designed equitably, and often, equity is only considered as an additional objective post hoc, which hampers systemic changes. We present a formulation for transit network design that considers different notions of equity and welfare explicitly. We study the interaction between network design and various concepts of equity and present trade-offs and results based on real-world data from a large metropolitan area in the United States of America.
- A. Zulqarnain, S. Gupta, J. P. Talusan, P. Pugliese, A. Mukhopadhyay, and A. Dubey, Addressing APC Data Sparsity in Predicting Occupancy and Delay of Transit Buses: A Multitask Learning Approach, in 2023 IEEE International Conference on Smart Computing (SMARTCOMP), 2023.
@inproceedings{Zulqarnain2023, author = {Zulqarnain, Ammar and Gupta, Samir and Talusan, Jose Paolo and Pugliese, Philip and Mukhopadhyay, Ayan and Dubey, Abhishek}, booktitle = {2023 IEEE International Conference on Smart Computing (SMARTCOMP)}, title = {Addressing APC Data Sparsity in Predicting Occupancy and Delay of Transit Buses: A Multitask Learning Approach}, year = {2023}, acceptance = {31}, contribution = {lead} }
Public transit is a vital mode of transportation in urban areas, and its efficiency is crucial for the daily commute of millions of people. To improve the reliability and predictability of transit systems, researchers have developed separate single-task learning models to predict the occupancy and delay of buses at the stop or route level. However, these models provide a narrow view of delay and occupancy at each stop and do not account for the correlation between the two. We propose a novel approach that leverages broader generalizable patterns governing delay and occupancy for improved prediction. We introduce a multitask learning toolchain that takes into account General Transit Feed Specification feeds, Automatic Passenger Counter data, and contextual information temporal and spatial information. The toolchain predicts transit delay and occupancy at the stop level, improving the accuracy of the predictions of these two features of a trip given sparse and noisy data. We also show that our toolchain can adapt to fewer samples of new transit data once it has been trained on previous routes/trips as compared to state-of-the-art methods. Finally, we use actual data from Chattanooga, Tennessee, to validate our approach. We compare our approach against the state-of-the-art methods and we show that treating occupancy and delay as related problems improves the accuracy of the predictions. We show that our approach improves delay prediction significantly by as much as 6% in F1 scores while producing equivalent or better results for occupancy.
- J. P. Talusan, A. Mukhopadhyay, D. Freudberg, and A. Dubey, On Designing Day Ahead and Same Day Ridership Level Prediction Models for City-Scale Transit Networks Using Noisy APC Data, in 2022 IEEE International Conference on Big Data (Big Data), Los Alamitos, CA, USA, 2022, pp. 5598–5606.
@inproceedings{talusan2022apc, author = {Talusan, Jose Paolo and Mukhopadhyay, Ayan and Freudberg, Dan and Dubey, Abhishek}, booktitle = {2022 IEEE International Conference on Big Data (Big Data)}, title = {On Designing Day Ahead and Same Day Ridership Level Prediction Models for City-Scale Transit Networks Using Noisy APC Data}, year = {2022}, address = {Los Alamitos, CA, USA}, month = dec, pages = {5598-5606}, publisher = {IEEE Computer Society}, contribution = {lead}, doi = {10.1109/BigData55660.2022.10020390}, keywords = {training;schedules;statistical analysis;stochastic processes;predictive models;big data;data models}, url = {https://doi.ieeecomputersociety.org/10.1109/BigData55660.2022.10020390} }
The ability to accurately predict public transit ridership demand benefits passengers and transit agencies. Agencies will be able to reallocate buses to handle under or over-utilized bus routes, improving resource utilization, and passengers will be able to adjust and plan their schedules to avoid overcrowded buses and maintain a certain level of comfort. However, accurately predicting occupancy is a non-trivial task. Various reasons such as heterogeneity, evolving ridership patterns, exogenous events like weather, and other stochastic variables, make the task much more challenging. With the progress of big data, transit authorities now have access to real-time passenger occupancy information for their vehicles. The amount of data generated is staggering. While there is no shortage in data, it must still be cleaned, processed, augmented, and merged before any useful information can be generated. In this paper, we propose the use and fusion of data from multiple sources, cleaned, processed, and merged together, for use in training machine learning models to predict transit ridership. We use data that spans a 2-year period (2020-2022) incorporating transit, weather, traffic, and calendar data. The resulting data, which equates to 17 million observations, is used to train separate models for the trip and stop level prediction. We evaluate our approach on real-world transit data provided by the public transit agency of Nashville, TN. We demonstrate that the trip level model based on Xgboost and the stop level model based on LSTM outperform the baseline statistical model across the entire transit service day.
- G. Pettet, A. Mukhopadhyay, M. J. Kochenderfer, and A. Dubey, Hierarchical Planning for Dynamic Resource Allocation in Smart and Connected Communities, ACM Trans. Cyber-Phys. Syst., vol. 6, no. 4, Nov. 2022.
@article{pettet2021hierarchical, author = {Pettet, Geoffrey and Mukhopadhyay, Ayan and Kochenderfer, Mykel J. and Dubey, Abhishek}, journal = {ACM Trans. Cyber-Phys. Syst.}, title = {Hierarchical Planning for Dynamic Resource Allocation in Smart and Connected Communities}, year = {2022}, issn = {2378-962X}, month = nov, number = {4}, volume = {6}, address = {New York, NY, USA}, articleno = {32}, contribution = {lead}, doi = {10.1145/3502869}, issue_date = {October 2022}, keywords = {planning under uncertainty, semi-Markov decision process, large-scale CPS, hierarchical planning, Dynamic resource allocation}, numpages = {26}, preprint = {https://arxiv.org/abs/2107.01292}, publisher = {Association for Computing Machinery}, url = {https://doi-org.proxy.library.vanderbilt.edu/10.1145/3502869} }
Resource allocation under uncertainty is a classic problem in city-scale cyber-physical systems. Consider emergency response, where urban planners and first responders optimize the location of ambulances to minimize expected response times to incidents such as road accidents. Typically, such problems involve sequential decision making under uncertainty and can be modeled as Markov (or semi-Markov) decision processes. The goal of the decision maker is to learn a mapping from states to actions that can maximize expected rewards. While online, offline, and decentralized approaches have been proposed to tackle such problems, scalability remains a challenge for real world use cases. We present a general approach to hierarchical planning that leverages structure in city level CPS problems for resource allocation. We use emergency response as a case study and show how a large resource allocation problem can be split into smaller problems. We then use Monte Carlo planning for solving the smaller problems and managing the interaction between them. Finally, we use data from Nashville, Tennessee, a major metropolitan area in the United States, to validate our approach. Our experiments show that the proposed approach outperforms state-of-the-art approaches used in the field of emergency response.
- S. Eisele, M. Wilbur, T. Eghtesad, K. Silvergold, F. Eisele, A. Mukhopadhyay, A. Laszka, and A. Dubey, Decentralized Computation Market for Stream Processing Applications, in 2022 IEEE International Conference on Cloud Engineering (IC2E), Pacific Grove, CA, USA, 2022.
@inproceedings{eisele2022Decentralized, author = {Eisele, Scott and Wilbur, Michael and Eghtesad, Taha and Silvergold, Kevin and Eisele, Fred and Mukhopadhyay, Ayan and Laszka, Aron and Dubey, Abhishek}, booktitle = {2022 IEEE International Conference on Cloud Engineering (IC2E)}, title = {Decentralized Computation Market for Stream Processing Applications}, year = {2022}, address = {Pacific Grove, CA, USA}, month = oct, acceptance = {32.6}, publisher = {IEEE Computer Society}, contribution = {lead} }
While cloud computing is the current standard for outsourcing computation, it can be prohibitively expensive for cities and infrastructure operators to deploy services. At the same time, there are underutilized computing resources within cities and local edge-computing deployments. Using these slack resources may enable significantly lower pricing than comparable cloud computing; such resources would incur minimal marginal expenditure since their deployment and operation are mostly sunk costs. However, there are challenges associated with using these resources. First, they are not effectively aggregated or provisioned. Second, there is a lack of trust between customers and suppliers of computing resources, given that they are distinct stakeholders and behave according to their own interests. Third, delays in processing inputs may diminish the value of the applications. To resolve these challenges, we introduce an architecture combining a distributed trusted computing mechanism, such as a blockchain, with an efficient messaging system like Apache Pulsar. Using this architecture, we design a decentralized computation market where customers and suppliers make offers to deploy and host applications. The proposed architecture can be realized using any trusted computing mechanism that supports smart contracts, and any messaging framework with the necessary features. This combination ensures that the market is robust without incurring the input processing delays that limit other blockchain based solutions. We evaluate the market protocol using game-theoretic analysis to show that deviation from the protocol is discouraged. Finally, we assess the performance of a prototype implementation based on experiments with a streaming computer-vision application.
- V. Nair, K. Prakash, M. Wilbur, A. Taneja, C. Namblard, O. Adeyemo, A. Dubey, A. Adereni, M. Tambe, and A. Mukhopadhyay, ADVISER: AI-Driven Vaccination Intervention Optimiser for Increasing Vaccine Uptake in Nigeria, in 31st International Joint Conference on Artificial Intelligence (IJCAI), 2022.
@inproceedings{ijcai22Ayan, author = {Nair, Vineet and Prakash, Kritika and Wilbur, Michael and Taneja, Aparna and Namblard, Corinne and Adeyemo, Oyindamola and Dubey, Abhishek and Adereni, Abiodun and Tambe, Milind and Mukhopadhyay, Ayan}, booktitle = {31st International Joint Conference on Artificial Intelligence (IJCAI)}, title = {ADVISER: AI-Driven Vaccination Intervention Optimiser for Increasing Vaccine Uptake in Nigeria}, year = {2022}, month = jul, acceptance = {15}, contribution = {minor}, doi = {https://doi.org/10.48550/ARXIV.2204.13663}, url = {https://arxiv.org/abs/2204.13663} }
More than 5 million children under five years die from largely preventable or treatable medical conditions every year, with an overwhelmingly large proportion of deaths occurring in under-developed countries with low vaccination uptake. One of the United Nations’ sustainable development goals (SDG 3) aims to end preventable deaths of newborns and children under five years of age. We focus on Nigeria, where the rate of infant mortality is appalling. We collaborate with HelpMum, a large non-profit organization in Nigeria to design and optimize the allocation of heterogeneous health interventions under uncertainty to increase vaccination uptake, the first such collaboration in Nigeria. Our framework, ADVISER: AI-Driven Vaccination Intervention Optimiser, is based on an integer linear program that seeks to maximize the cumulative probability of successful vaccination. Our optimization formulation is intractable in practice. We present a heuristic approach that enables us to solve the problem for real-world use-cases. We also present theoretical bounds for the heuristic method. Finally, we show that the proposed approach outperforms baseline methods in terms of vaccination uptake through experimental evaluation. HelpMum is currently planning a pilot program based on our approach to be deployed in the largest city of Nigeria, which would be the first deployment of an AIdriven vaccination uptake program in the country and hopefully, pave the way for other data-driven programs to improve health outcomes in Nigeria.
- A. Sivagnanam, S. U. Kadir, A. Mukhopadhyay, P. Pugliese, A. Dubey, S. Samaranayake, and A. Laszka, Offline Vehicle Routing Problem with Online Bookings: A Novel Problem Formulation with Applications to Paratransit, in 31st International Joint Conference on Artificial Intelligence (IJCAI), 2022.
@inproceedings{sivagnanam2022offline, author = {Sivagnanam, Amutheezan and Kadir, Salah Uddin and Mukhopadhyay, Ayan and Pugliese, Philip and Dubey, Abhishek and Samaranayake, Samitha and Laszka, Aron}, booktitle = {31st International Joint Conference on Artificial Intelligence (IJCAI)}, title = {Offline Vehicle Routing Problem with Online Bookings: A Novel Problem Formulation with Applications to Paratransit}, year = {2022}, acceptance = {15}, month = jul, contribution = {colab}, preprint = {https://arxiv.org/abs/2204.11992} }
Vehicle routing problems (VRPs) can be divided into two major categories: offline VRPs, which consider a given set of trip requests to be served, and online VRPs, which consider requests as they arrive in real-time. Based on discussions with public transit agencies, we identify a real-world problem that is not addressed by existing formulations: booking trips with flexible pickup windows (e.g., 3 hours) in advance (e.g., the day before) and confirming tight pickup windows (e.g., 30 minutes) at the time of booking. Such a service model is often required in paratransit service settings, where passengers typically book trips for the next day over the phone. To address this gap between offline and online problems, we introduce a novel formulation, the offline vehicle routing problem with online bookings. This problem is very challenging computationally since it faces the complexity of considering large sets of requests—similar to offline VRPs—but must abide by strict constraints on running time—similar to online VRPs. To solve this problem, we propose a novel computational approach, which combines an anytime algorithm with a learning-based policy for real-time decisions. Based on a paratransit dataset obtained from our partner transit agency, we demonstrate that our novel formulation and computational approach lead to significantly better outcomes in this service setting than existing algorithms.
- G. Pettet, H. Baxter, S. Vazirizade, H. Purohit, M. Ma, A. Mukhopadhyay, and A. Dubey, Designing Decision Support Systems for Emergency Response: Challenges and Opportunities, in 2022 Workshop on Cyber Physical Systems for Emergency Response (CPS-ER), Los Alamitos, CA, USA, 2022, pp. 30–35.
@inproceedings{pettet2022designing, author = {Pettet, G. and Baxter, H. and Vazirizade, S. and Purohit, H. and Ma, M. and Mukhopadhyay, A. and Dubey, A.}, booktitle = {2022 Workshop on Cyber Physical Systems for Emergency Response (CPS-ER)}, title = {Designing Decision Support Systems for Emergency Response: Challenges and Opportunities}, year = {2022}, address = {Los Alamitos, CA, USA}, month = may, pages = {30-35}, publisher = {IEEE Computer Society}, contribution = {lead}, doi = {10.1109/CPS-ER56134.2022.00012}, keywords = {decision support systems;road accidents;uncertainty;decision making;medical services;emergency services;hazards}, url = {https://doi.ieeecomputersociety.org/10.1109/CPS-ER56134.2022.00012} }
Designing effective emergency response management (ERM) systems to respond to incidents such as road accidents is a major problem faced by communities. In addition to responding to frequent incidents each day (about 240 million emergency medical services calls and over 5 million road accidents in the US each year), these systems also support response during natural hazards. Recently, there has been a consistent interest in building decision support and optimization tools that can help emergency responders provide more efficient and effective response. This includes a number of principled subsystems that implement early incident detection, incident likelihood forecasting and strategic resource allocation and dispatch policies. In this paper, we highlight the key challenges and provide an overview of the approach developed by our team in collaboration with our community partners.
- M. Wilbur, S. Kadir, Y. Kim, G. Pettet, A. Mukhopadhyay, P. Pugliese, S. Samaranayake, A. Laszka, and A. Dubey, An Online Approach to Solve the Dynamic Vehicle Routing Problem with Stochastic Trip Requests for Paratransit Services, in ACM/IEEE 13th International Conference on Cyber-Physical Systems (ICCPS), 2022.
@inproceedings{wilbur2022, author = {Wilbur, Michael and Kadir, Salah and Kim, Youngseo and Pettet, Geoffrey and Mukhopadhyay, Ayan and Pugliese, Philip and Samaranayake, Samitha and Laszka, Aron and Dubey, Abhishek}, booktitle = {ACM/IEEE 13th International Conference on Cyber-Physical Systems (ICCPS)}, title = {An Online Approach to Solve the Dynamic Vehicle Routing Problem with Stochastic Trip Requests for Paratransit Services}, year = {2022}, month = apr, acceptance = {28}, publisher = {IEEE}, contribution = {lead} }
Many transit agencies operating paratransit and microtransit services have to respond to trip requests that arrive in real-time, which entails solving hard combinatorial and sequential decision-making problems under uncertainty. To avoid decisions that lead to significant inefficiency in the long term, vehicles should be allocated to requests by optimizing a non-myopic utility function or by batching requests together and optimizing a myopic utility function. While the former approach is typically offline, the latter can be performed online. We point out two major issues with such approaches when applied to paratransit services in practice. First, it is difficult to batch paratransit requests together as they are temporally sparse. Second, the environment in which transit agencies operate changes dynamically (e.g., traffic conditions can change over time), causing the estimates that are learned offline to become stale. To address these challenges, we propose a fully online approach to solve the dynamic vehicle routing problem (DVRP) with time windows and stochastic trip requests that is robust to changing environmental dynamics by construction. We focus on scenarios where requests are relatively sparse—our problem is motivated by applications to paratransit services. We formulate DVRP as a Markov decision process and use Monte Carlo tree search to compute near-optimal actions for any given state. Accounting for stochastic requests while optimizing a non-myopic utility function is computationally challenging; indeed, the action space for such a problem is intractably large in practice. To tackle the large action space, we leverage the structure of the problem to design heuristics that can sample promising actions for the tree search. Our experiments using real-world data from our partner agency show that the proposed approach outperforms existing state-of-the-art approaches both in terms of performance and robustness.
- Z. Kang, A. Mukhopadhyay, A. Gokhale, S. Wen, and A. Dubey, Traffic Anomaly Detection Via Conditional Normalizing Flow, in 2022 IEEE 25th International Conference on Intelligent Transportation Systems (ITSC), 2022, pp. 2563–2570.
@inproceedings{kang2022generative, author = {Kang, Zhuangwei and Mukhopadhyay, Ayan and Gokhale, Aniruddha and Wen, Shijie and Dubey, Abhishek}, booktitle = {2022 IEEE 25th International Conference on Intelligent Transportation Systems (ITSC)}, title = {Traffic Anomaly Detection Via Conditional Normalizing Flow}, year = {2022}, pages = {2563-2570}, contribution = {lead}, doi = {10.1109/ITSC55140.2022.9922061} }
Traffic congestion anomaly detection is of paramount importance in intelligent traffic systems. The goals of transportation agencies are two-fold: to monitor the general traffic conditions in the area of interest and to locate road segments under abnormal congestion states. Modeling congestion patterns can achieve these goals for citywide roadways, which amounts to learning the distribution of multivariate time series (MTS). However, existing works are either not scalable or unable to capture the spatial-temporal information in MTS simultaneously. To this end, we propose a principled and comprehensive framework consisting of a data-driven generative approach that can perform tractable density estimation for detecting traffic anomalies. Our approach first clusters segments in the feature space and then uses conditional normalizing flow to identify anomalous temporal snapshots at the cluster level in an unsupervised setting. Then, we identify anomalies at the segment level by using a kernel density estimator on the anomalous cluster. Extensive experiments on synthetic datasets show that our approach significantly outperforms several state-of-the-art congestion anomaly detection and diagnosis methods in terms of Recall and F1-Score. We also use the generative model to sample labeled data, which can train classifiers in a supervised setting, alleviating the lack of labeled data for anomaly detection in sparse settings.
- A. Mukhopadhyay, G. Pettet, S. M. Vazirizade, D. Lu, A. Jaimes, S. E. Said, H. Baroud, Y. Vorobeychik, M. Kochenderfer, and A. Dubey, A Review of Incident Prediction, Resource Allocation, and Dispatch Models for Emergency Management, Accident Analysis & Prevention, vol. 165, p. 106501, 2022.
@article{mukhopadhyay2021review, author = {Mukhopadhyay, Ayan and Pettet, Geoffrey and Vazirizade, Sayyed Mohsen and Lu, Di and Jaimes, Alejandro and Said, Said El and Baroud, Hiba and Vorobeychik, Yevgeniy and Kochenderfer, Mykel and Dubey, Abhishek}, journal = {Accident Analysis & Prevention}, title = {A Review of Incident Prediction, Resource Allocation, and Dispatch Models for Emergency Management}, year = {2022}, issn = {0001-4575}, pages = {106501}, volume = {165}, contribution = {lead}, doi = {https://doi.org/10.1016/j.aap.2021.106501}, keywords = {Resource allocation for smart cities, Incident prediction, Computer aided dispatch, Decision making under uncertainty, Accident analysis, Emergency response}, preprint = {https://arxiv.org/abs/2006.04200}, url = {https://www.sciencedirect.com/science/article/pii/S0001457521005327} }
In the last fifty years, researchers have developed statistical, data-driven, analytical, and algorithmic approaches for designing and improving emergency response management (ERM) systems. The problem has been noted as inherently difficult and constitutes spatio-temporal decision making under uncertainty, which has been addressed in the literature with varying assumptions and approaches. This survey provides a detailed review of these approaches, focusing on the key challenges and issues regarding four sub-processes: (a) incident prediction, (b) incident detection, (c) resource allocation, and (c) computer-aided dispatch for emergency response. We highlight the strengths and weaknesses of prior work in this domain and explore the similarities and differences between different modeling paradigms. We conclude by illustrating open challenges and opportunities for future research in this complex domain.
- G. Pettet, A. Mukhopadhyay, and A. Dubey, Decision Making in Non-Stationary Environments with Policy-Augmented Monte Carlo Tree Search. 2022.
@misc{pettet2022decision, author = {Pettet, Geoffrey and Mukhopadhyay, Ayan and Dubey, Abhishek}, title = {Decision Making in Non-Stationary Environments with Policy-Augmented Monte Carlo Tree Search}, year = {2022}, archiveprefix = {arXiv}, contribution = {lead}, eprint = {2202.13003}, preprint = {https://arxiv.org/abs/2202.13003}, primaryclass = {cs.AI} }
Decision-making under uncertainty (DMU) is present in many important problems. An open challenge is DMU in non-stationary environments, where the dynamics of the environment can change over time. Reinforcement Learning (RL), a popular approach for DMU problems, learns a policy by interacting with a model of the environment offline. Unfortunately, if the environment changes the policy can become stale and take sub-optimal actions, and relearning the policy for the updated environment takes time and computational effort. An alternative is online planning approaches such as Monte Carlo Tree Search (MCTS), which perform their computation at decision time. Given the current environment, MCTS plans using high-fidelity models to determine promising action trajectories. These models can be updated as soon as environmental changes are detected to immediately incorporate them into decision making. However, MCTS’s convergence can be slow for domains with large state-action spaces. In this paper, we present a novel hybrid decision-making approach that combines the strengths of RL and planning while mitigating their weaknesses. Our approach, called Policy Augmented MCTS (PA-MCTS), integrates a policy’s actin-value estimates into MCTS, using the estimates to seed the action trajectories favored by the search. We hypothesize that PA-MCTS will converge more quickly than standard MCTS while making better decisions than the policy can make on its own when faced with nonstationary environments. We test our hypothesis by comparing PA-MCTS with pure MCTS and an RL agent applied to the classical CartPole environment. We find that PC-MCTS can achieve higher cumulative rewards than the policy in isolation under several environmental shifts while converging in significantly fewer iterations than pure MCTS.
- S. M. Vazirizade, A. Mukhopadhyay, G. Pettet, S. El Said, H. Baroud, and A. Dubey, Learning Incident Prediction Models Over Large Geographical Areas for Emergency Response, in 2021 IEEE International Conference on Smart Computing (SMARTCOMP), 2021, pp. 424–429.
@inproceedings{vazirizade2021learning, author = {Vazirizade, Sayyed Mohsen and Mukhopadhyay, Ayan and Pettet, Geoffrey and El Said, Said and Baroud, Hiba and Dubey, Abhishek}, booktitle = {2021 IEEE International Conference on Smart Computing (SMARTCOMP)}, title = {Learning Incident Prediction Models Over Large Geographical Areas for Emergency Response}, year = {2021}, month = aug, pages = {424-429}, acceptance = {31.7}, contribution = {lead}, doi = {10.1109/SMARTCOMP52413.2021.00091}, issn = {2693-8340}, keywords = {Road accidents;Pipelines;Collaboration;Weather forecasting;Predictive models;Emergency services;Resource management;Spatial Temporal Incident Prediction;Emergency Response Management;Resource Allocation;Statistical Modeling}, tag = {ai4cps,incident} }
Emergency Response Management (ERM) necessitates the use of models capable of predicting the spatial-temporal likelihood of incident occurrence. These models are used for proactive stationing in order to reduce overall response time. Traditional methods simply aggregate past incidents over space and time; such approaches fail to make useful short-term predictions when the spatial region is large and focused on fine-grained spatial entities like interstate highway networks. This is partially due to the sparsity of incidents with respect to space and time. Further, accidents are affected by several covariates. Collecting, cleaning, and managing multiple streams of data from various sources is challenging for large spatial areas. In this paper, we highlight how this problem is being solved in collaboration with the Tennessee Department of Transportation (TDOT) to improve ERM in the state of Tennessee. Our pipeline, based on a combination of synthetic resampling, clustering, and data mining techniques, can efficiently forecast the spatio-temporal dynamics of accident occurrence, even under sparse conditions. Our pipeline uses data related to roadway geometry, weather, historical accidents, and traffic to aid accident forecasting. To understand how our forecasting model can affect allocation and dispatch, we improve and employ a classical resource allocation approach. Experimental results show that our approach can noticeably reduce response times and the number of unattended incidents in comparison to current approaches followed by first responders. The developed pipeline is efficacious, applicable in practice, and open-source.
- M. Wilbur, A. Mukhopadhyay, S. Vazirizade, P. Pugliese, A. Laszka, and A. Dubey, Energy and Emission Prediction for Mixed-Vehicle Transit Fleets Using Multi-Task and Inductive Transfer Learning, in Joint European Conference on Machine Learning and Knowledge Discovery in Databases, 2021.
@inproceedings{ecml2021, author = {Wilbur, Michael and Mukhopadhyay, Ayan and Vazirizade, Sayyed and Pugliese, Philip and Laszka, Aron and Dubey, Abhishek}, booktitle = {Joint European Conference on Machine Learning and Knowledge Discovery in Databases}, title = {Energy and Emission Prediction for Mixed-Vehicle Transit Fleets Using Multi-Task and Inductive Transfer Learning}, year = {2021}, acceptance = {29}, contribution = {lead}, tag = {ai4cps,transit} }
Public transit agencies are focused on making their fixed-line bus systems more energy efficient by introducing electric (EV) and hybrid (HV) vehicles to their eets. However, because of the high upfront cost of these vehicles, most agencies are tasked with managing a mixed-fleet of internal combustion vehicles (ICEVs), EVs, and HVs. In managing mixed-fleets, agencies require accurate predictions of energy use for optimizing the assignment of vehicles to transit routes, scheduling charging, and ensuring that emission standards are met. The current state-of-the-art is to develop separate neural network models to predict energy consumption for each vehicle class. Although different vehicle classes’ energy consumption depends on a varied set of covariates, we hypothesize that there are broader generalizable patterns that govern energy consumption and emissions. In this paper, we seek to extract these patterns to aid learning to address two problems faced by transit agencies. First, in the case of a transit agency which operates many ICEVs, HVs, and EVs, we use multi-task learning (MTL) to improve accuracy of forecasting energy consumption. Second, in the case where there is a significant variation in vehicles in each category, we use inductive transfer learning (ITL) to improve predictive accuracy for vehicle class models with insufficient data. As this work is to be deployed by our partner agency, we also provide an online pipeline for joining the various sensor streams for fixed-line transit energy prediction. We find that our approach outperforms vehicle-specific baselines in both the MTL and ITL settings.
- G. Pettet, A. Mukhopadhyay, M. Kochenderfer, and A. Dubey, Hierarchical Planning for Resource Allocation in Emergency Response Systems, in Proceedings of the 12th ACM/IEEE International Conference on Cyber-Physical Systems, ICCPS 2021, Nashville, TN, USA, 2021.
@inproceedings{iccps2021, author = {Pettet, Geoffrey and Mukhopadhyay, Ayan and Kochenderfer, Mykel and Dubey, Abhishek}, booktitle = {Proceedings of the 12th {ACM/IEEE} International Conference on Cyber-Physical Systems, {ICCPS} 2021, Nashville, TN, USA}, title = {Hierarchical Planning for Resource Allocation in Emergency Response Systems}, year = {2021}, acceptance = {26}, contribution = {lead}, keywords = {emergency}, project = {smart-cities,smart-emergency-response}, tag = {ai4cps,decentralization,incident} }
A classical problem in city-scale cyber-physical systems (CPS) is resource allocation under uncertainty. Spatial-temporal allocation of resources is optimized to allocate electric scooters across urban areas, place charging stations for vehicles, and design efficient on-demand transit. Typically, such problems are modeled as Markov (or semi-Markov) decision processes. While online, offline, and decentralized methodologies have been used to tackle such problems, none of the approaches scale well for large-scale decision problems. We create a general approach to hierarchical planning that leverages structure in city-level CPS problems to tackle resource allocation under uncertainty. We use emergency response as a case study and show how a large resource allocation problem can be split into smaller problems. We then create a principled framework for solving the smaller problems and tackling the interaction between them. Finally, we use real-world data from a major metropolitan area in the United States to validate our approach. Our experiments show that the proposed approach outperforms state-of-the-art approaches used in the field of emergency response.
- Y. Senarath, A. Mukhopadhyay, S. Vazirizade, hemant Purohit, S. Nannapaneni, and A. Dubey, Practitioner-Centric Approach for Early Incident Detection Using Crowdsourced Data for Emergency Services, in 21st IEEE International Conference on Data Mining (ICDM 2021), 2021.
@inproceedings{ICDM_2021, author = {Senarath, Yasas and Mukhopadhyay, Ayan and Vazirizade, Sayyed and hemant Purohit and Nannapaneni, Saideep and Dubey, Abhishek}, booktitle = {21st IEEE International Conference on Data Mining (ICDM 2021)}, title = {Practitioner-Centric Approach for Early Incident Detection Using Crowdsourced Data for Emergency Services}, year = {2021}, acceptance = {20}, contribution = {colab}, tag = {ai4cps,incident} }
Emergency response is highly dependent on the time of incident reporting. Unfortunately, the traditional approach to receiving incident reports (e.g., calling 911 in the USA) has time delays. Crowdsourcing platforms such as Waze provide an opportunity for early identification of incidents. However, detecting incidents from crowdsourced data streams is difficult due to the challenges of noise and uncertainty associated with such data. Further, simply optimizing over detection accuracy can compromise spatial-temporal localization of the inference, thereby making such approaches infeasible for real-world deployment. This paper presents a novel problem formulation and solution approach for practitioner-centered incident detection using crowdsourced data by using emergency response management as a case-study. The proposed approach CROME (Crowdsourced Multi-objective Event Detection) quantifies the relationship between the performance metrics of incident classification (e.g., F1 score) and the requirements of model practitioners (e.g., 1 km. radius for incident detection). First, we show how crowdsourced reports, ground-truth historical data, and other relevant determinants such as traffic and weather can be used together in a Convolutional Neural Network (CNN) architecture for early detection of emergency incidents. Then, we use a Pareto optimization-based approach to optimize the output of the CNN in tandem with practitioner-centric parameters to balance detection accuracy and spatial-temporal localization. Finally, we demonstrate the applicability of this approach using crowdsourced data from Waze and traffic accident reports from Nashville, TN, USA. Our experiments demonstrate that the proposed approach outperforms existing approaches in incident detection while simultaneously optimizing the needs for realworld deployment and usability.
- J. Martinez, A. M. A. Ayman, M. Wilbur, P. Pugliese, D. Freudberg, A. Laszka, and A. Dubey, Predicting Public Transportation Load to Estimate the Probability of Social Distancing Violations, in Proceedings of the Workshop on AI for Urban Mobility at the 35th AAAI Conference on Artificial Intelligence (AAAI-21), 2021.
@inproceedings{juan21, author = {Martinez, Juan and Ayman, Ayan Mukhopadhyay Afiya and Wilbur, Michael and Pugliese, Philip and Freudberg, Dan and Laszka, Aron and Dubey, Abhishek}, booktitle = {Proceedings of the Workshop on AI for Urban Mobility at the 35th AAAI Conference on Artificial Intelligence (AAAI-21)}, title = {Predicting Public Transportation Load to Estimate the Probability of Social Distancing Violations}, year = {2021}, contribution = {minor}, tag = {transit} }
Public transit agencies struggle to maintain transit accessibility with reduced resources, unreliable ridership data, reduced vehicle capacities due to social distancing, and reduced services due to driver unavailability. In collaboration with transit agencies from two large metropolitan areas in the USA, we are designing novel approaches for addressing the afore-mentioned challenges by collecting accurate real-time ridership data, providing guidance to commuters, and performing operational optimization for public transit. We estimate rider-ship data using historical automated passenger counting data, conditional on a set of relevant determinants. Accurate ridership forecasting is essential to optimize the public transit schedule, which is necessary to improve current fixed lines with on-demand transit. Also, passenger crowding has been a problem for public transportation since it deteriorates passengers’ wellbeing and satisfaction. During the COVID-19 pandemic, passenger crowding has gained importance since it represents a risk for social distancing violations. Therefore, we are creating optimization models to ensure that social distancing norms can be adequately followed while ensuring that the total demand for transit is met. We will then use accurate forecasts for operational optimization that includes (a) proactive fixed-line schedule optimization based on predicted demand, (b) dispatch of on-demand micro-transit, prioritizing at-risk populations, and (c) allocation of vehicles to transit and cargo trips, considering exigent vehicle maintenance requirements (i.e., disinfection). Finally, this paper presents some initial results from our project regarding the estimation of ridership in public transit.
- S. Singla, A. Mukhopadhyay, M. Wilbur, T. Diao, V. Gajjewar, A. Eldawy, M. Kochenderfer, R. Shachter, and A. Dubey, WildfireDB: An Open-Source Dataset ConnectingWildfire Spread with Relevant Determinants, in 35th Conference on Neural Information Processing Systems (NeurIPS 2021) Track on Datasets and Benchmarks, 2021.
@inproceedings{wildfiredb2021, author = {Singla, Samriddhi and Mukhopadhyay, Ayan and Wilbur, Michael and Diao, Tina and Gajjewar, Vinayak and Eldawy, Ahmed and Kochenderfer, Mykel and Shachter, Ross and Dubey, Abhishek}, booktitle = {35th Conference on Neural Information Processing Systems (NeurIPS 2021) Track on Datasets and Benchmarks}, title = {WildfireDB: An Open-Source Dataset ConnectingWildfire Spread with Relevant Determinants}, year = {2021}, acceptance = {26}, contribution = {minor}, tag = {ai4cps,incident} }
Modeling fire spread is critical in fire risk management. Creating data-driven models to forecast spread remains challenging due to the lack of comprehensive data sources that relate fires with relevant covariates. We present the first comprehensive and open-source dataset that relates historical fire data with relevant covariates such as weather, vegetation, and topography. Our dataset, named WildfireDB, contains over 17 million data points that capture how fires spread in the continental USA in the last decade. In this paper, we describe the algorithmic approach used to create and integrate the data, describe the dataset, and present benchmark results regarding data-driven models that can be learned to forecast the spread of wildfires.
- G. Pettet, A. Mukhopadhyay, M. Kochenderfer, Y. Vorobeychik, and A. Dubey, On Algorithmic Decision Procedures in Emergency Response Systems in Smart and Connected Communities, in Proceedings of the 19th Conference on Autonomous Agents and MultiAgent Systems, AAMAS 2020, Auckland, New Zealand, 2020.
@inproceedings{Pettet2020, author = {Pettet, Geoffrey and Mukhopadhyay, Ayan and Kochenderfer, Mykel and Vorobeychik, Yevgeniy and Dubey, Abhishek}, booktitle = {Proceedings of the 19th Conference on Autonomous Agents and MultiAgent Systems, {AAMAS} 2020, Auckland, New Zealand}, title = {On Algorithmic Decision Procedures in Emergency Response Systems in Smart and Connected Communities}, year = {2020}, category = {selectiveconference}, contribution = {lead}, acceptance = {23}, keywords = {emergency, performance}, project = {smart-emergency-response,smart-cities}, tag = {ai4cps, decentralization,incident}, timestamp = {Wed, 17 Jan 2020 07:24:00 +0200} }
Emergency Response Management (ERM) is a critical problem faced by communities across the globe. Despite its importance, it is common for ERM systems to follow myopic and straight-forward decision policies in the real world. Principled approaches to aid decision-making under uncertainty have been explored in this context but have failed to be accepted into real systems. We identify a key issue impeding their adoption — algorithmic approaches to emergency response focus on reactive, post-incident dispatching actions, i.e. optimally dispatching a responder after incidents occur. However, the critical nature of emergency response dictates that when an incident occurs, first responders always dispatch the closest available responder to the incident. We argue that the crucial period of planning for ERM systems is not post-incident, but between incidents. However, this is not a trivial planning problem - a major challenge with dynamically balancing the spatial distribution of responders is the complexity of the problem. An orthogonal problem in ERM systems is to plan under limited communication, which is particularly important in disaster scenarios that affect communication networks. We address both the problems by proposing two partially decentralized multi-agent planning algorithms that utilize heuristics and the structure of the dispatch problem. We evaluate our proposed approach using real-world data, and find that in several contexts, dynamic re-balancing the spatial distribution of emergency responders reduces both the average response time as well as its variance.
- A. Mukhopadhyay, G. Pettet, C. Samal, A. Dubey, and Y. Vorobeychik, An online decision-theoretic pipeline for responder dispatch, in Proceedings of the 10th ACM/IEEE International Conference on Cyber-Physical Systems, ICCPS 2019, Montreal, QC, Canada, 2019, pp. 185–196.
@inproceedings{Mukhopadhyay2019, author = {Mukhopadhyay, Ayan and Pettet, Geoffrey and Samal, Chinmaya and Dubey, Abhishek and Vorobeychik, Yevgeniy}, booktitle = {Proceedings of the 10th {ACM/IEEE} International Conference on Cyber-Physical Systems, {ICCPS} 2019, Montreal, QC, Canada}, title = {An online decision-theoretic pipeline for responder dispatch}, year = {2019}, pages = {185--196}, bibsource = {dblp computer science bibliography, https://dblp.org}, biburl = {https://dblp.org/rec/bib/conf/iccps/MukhopadhyayPSD19}, category = {selectiveconference}, contribution = {lead}, acceptance = {27}, doi = {10.1145/3302509.3311055}, file = {:Mukhopadhyay2019-An_Online_Decision_Theoretic_Pipeline_for_Responder_Dispatch.pdf:PDF}, keywords = {emergency}, project = {smart-cities,smart-emergency-response}, tag = {ai4cps,incident}, timestamp = {Sun, 07 Apr 2019 16:25:36 +0200}, url = {https://doi.org/10.1145/3302509.3311055} }
The problem of dispatching emergency responders to service traffic accidents, fire, distress calls and crimes plagues urban areas across the globe. While such problems have been extensively looked at, most approaches are offline. Such methodologies fail to capture the dynamically changing environments under which critical emergency response occurs, and therefore, fail to be implemented in practice. Any holistic approach towards creating a pipeline for effective emergency response must also look at other challenges that it subsumes - predicting when and where incidents happen and understanding the changing environmental dynamics. We describe a system that collectively deals with all these problems in an online manner, meaning that the models get updated with streaming data sources. We highlight why such an approach is crucial to the effectiveness of emergency response, and present an algorithmic framework that can compute promising actions for a given decision-theoretic model for responder dispatch. We argue that carefully crafted heuristic measures can balance the trade-off between computational time and the quality of solutions achieved and highlight why such an approach is more scalable and tractable than traditional approaches. We also present an online mechanism for incident prediction, as well as an approach based on recurrent neural networks for learning and predicting environmental features that affect responder dispatch. We compare our methodology with prior state-of-the-art and existing dispatch strategies in the field, which show that our approach results in a reduction in response time with a drastic reduction in computational time.
- G. Pettet, A. Mukhopadhyay, C. Samal, A. Dubey, and Y. Vorobeychik, Incident management and analysis dashboard for fire departments: ICCPS demo, in Proceedings of the 10th ACM/IEEE International Conference on Cyber-Physical Systems, ICCPS 2019, Montreal, QC, Canada, 2019, pp. 336–337.
@inproceedings{Pettet2019, author = {Pettet, Geoffrey and Mukhopadhyay, Ayan and Samal, Chinmaya and Dubey, Abhishek and Vorobeychik, Yevgeniy}, booktitle = {Proceedings of the 10th {ACM/IEEE} International Conference on Cyber-Physical Systems, {ICCPS} 2019, Montreal, QC, Canada}, title = {Incident management and analysis dashboard for fire departments: {ICCPS} demo}, year = {2019}, pages = {336--337}, bibsource = {dblp computer science bibliography, https://dblp.org}, biburl = {https://dblp.org/rec/bib/conf/iccps/PettetMSDV19}, category = {poster}, contribution = {lead}, doi = {10.1145/3302509.3313329}, file = {:Pettet2019-Incident_management_and_analysis_dashboard_for_fire_departments_ICCPS_demo.pdf:PDF}, keywords = {emergency}, project = {smart-cities,smart-emergency-response}, tag = {incident}, timestamp = {Sun, 07 Apr 2019 16:25:36 +0200}, url = {https://doi.org/10.1145/3302509.3313329} }
This work presents a dashboard tool that helps emergency responders analyze and manage spatial-temporal incidents like crime and traffic accidents. It uses state-of-the-art statistical models to learn incident probabilities based on factors such as prior incidents, time and weather. The dashboard can then present historic and predicted incident distributions. It also allows responders to analyze how moving or adding depots (stations for emergency responders) affects average response times, and can make dispatching recommendations based on heuristics. Broadly, it is a one-stop tool that helps responders visualize historical data as well as plan for and respond to incidents.
- A. Mukhopadhyay, Y. Vorobeychik, A. Dubey, and G. Biswas, Prioritized Allocation of Emergency Responders based on a Continuous-Time Incident Prediction Model, in Proceedings of the 16th Conference on Autonomous Agents and MultiAgent Systems, AAMAS 2017, São Paulo, Brazil, May 8-12, 2017, 2017, pp. 168–177.
@inproceedings{Mukhopadhyay2017, author = {Mukhopadhyay, Ayan and Vorobeychik, Yevgeniy and Dubey, Abhishek and Biswas, Gautam}, booktitle = {Proceedings of the 16th Conference on Autonomous Agents and MultiAgent Systems, {AAMAS} 2017, S{\~{a}}o Paulo, Brazil, May 8-12, 2017}, title = {Prioritized Allocation of Emergency Responders based on a Continuous-Time Incident Prediction Model}, year = {2017}, acceptance = {27}, pages = {168--177}, bibsource = {dblp computer science bibliography, https://dblp.org}, biburl = {https://dblp.org/rec/bib/conf/atal/MukhopadhyayVDB17}, category = {selectiveconference}, contribution = {colab}, file = {:Mukhopadhyay2017-Prioritized_Allocation_of_Emergency_Responders_based_on_a_Continuous-Time_Incident_Prediction_Model.pdf:PDF}, keywords = {emergency}, project = {smart-emergency-response,smart-cities}, tag = {ai4cps,incident}, timestamp = {Wed, 27 Sep 2017 07:24:00 +0200}, url = {http://dl.acm.org/citation.cfm?id=3091154} }
Efficient emergency response is a major concern in densely populated urban areas. Numerous techniques have been proposed to allocate emergency responders to optimize response times, coverage, and incident prevention. Effective response depends, in turn, on effective prediction of incidents occurring in space and time, a problem which has also received considerable prior attention. We formulate a non-linear mathematical program maximizing expected incident coverage, and propose a novel algorithmic framework for solving this problem. In order to aid the optimization problem, we propose a novel incident prediction mechanism. Prior art in incident prediction does not generally consider incident priorities which are crucial in optimal dispatch, and spatial modeling either considers each discretized area independently, or learns a homogeneous model. We bridge these gaps by learning a joint distribution of both incident arrival time and severity, with spatial heterogeneity captured using a hierarchical clustering approach. Moreover, our decomposition of the joint arrival and severity distributions allows us to independently learn the continuous-time arrival model, and subsequently use a multinomial logistic regression to capture severity, conditional on incident time. We use real traffic accident and response data from the urban area around Nashville, USA, to evaluate the proposed approach, showing that it significantly outperforms prior art as well as the real dispatch method currently in use.
Ayan’s full publication list is available here